舉報
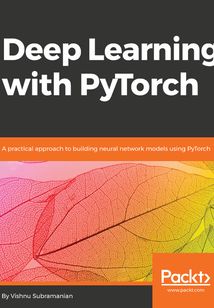
會員
Deep Learning with PyTorch
Thisbookisformachinelearningengineers,dataanalysts,datascientistsinterestedindeeplearningandarelookingtoexploreimplementingadvancedalgorithmsinPyTorch.Someknowledgeofmachinelearningishelpfulbutnotamandatoryneed.WorkingknowledgeofPythonprogrammingisexpected.
最新章節(jié)
- Leave a review - let other readers know what you think
- Other Books You May Enjoy
- Summary
- How to keep yourself updated
- Open Neural Network Exchange
- fast.ai – making neural nets uncool again
品牌:中圖公司
上架時間:2021-06-24 18:03:09
出版社:Packt Publishing
本書數(shù)字版權由中圖公司提供,并由其授權上海閱文信息技術有限公司制作發(fā)行
- Leave a review - let other readers know what you think 更新時間:2021-06-24 19:17:02
- Other Books You May Enjoy
- Summary
- How to keep yourself updated
- Open Neural Network Exchange
- fast.ai – making neural nets uncool again
- Alien NLP
- OpenNMT in PyTorch
- Image segmentation
- Object detection
- Interesting ideas to explore
- Overview
- What next?
- What Next?
- Summary
- Decoder
- Encoder
- Encoder-decoder architecture
- Training and validating the model
- Creating an ensembling model
- Creating a custom dataset along with data loaders
- Extracting the image features
- Creating models
- Model ensembling
- Creating a fully connected model and train
- Creating a dataset and loaders
- Extracting DenseNet features
- Creating a DenseNet model
- DenseLayer
- DenseBlock
- Densely connected convolutional networks – DenseNet
- Training and validating the model
- Creating a fully connected model
- Creating a new dataset for the convoluted features
- Extracting convolutional features using register_forward_hook
- Creating an Inception model
- Inception
- Training and validating the model
- Creating a simple linear model
- Creating a custom PyTorch dataset class for the pre-convoluted features and loader
- Extracting convolutional features
- Creating a ResNet model
- Creating loaders for training and validation
- Creating PyTorch datasets
- ResNet
- Modern network architectures
- Modern Network Architectures
- Summary
- Training the model
- Defining the train and evaluate functions
- Defining a model based on LSTM
- Backpropagation through time
- Batches
- Generating the batches
- Preparing the data
- Language modeling
- Inspecting the generated images
- Training the complete network
- Training the generator network
- Training the discriminator with fake images
- Training the discriminator with real images
- Training the discriminator
- Defining loss and optimizer
- Defining the discriminator network
- Generator
- Batch normalization
- Transposed convolutions
- Defining the generator network
- Deep convolutional GAN
- Generative adversarial networks
- Training
- Creating the optimizer
- Creating loss function for each layers
- Extracting the losses
- Style loss
- Content loss
- Creating the VGG model
- Loading the data
- Neural style transfer
- Generative Networks
- Summary
- Training the model
- Creating the network
- Understanding one-dimensional convolution for sequence data
- Convolutional network on sequence data
- Training the model
- Creating the network
- Creating batches
- Preparing the data
- LSTM networks
- Long-term dependency
- LSTM
- Understanding how RNN works with an example
- Recursive neural networks
- Freeze the embedding layer weights
- Loading the embeddings in the model
- Downloading the embeddings
- Using pretrained word embeddings
- Training the model
- Creating a network model with embedding
- Generate batches of vectors
- Building vocabulary
- torchtext.datasets
- torchtext.data
- Downloading IMDB data and performing text tokenization
- Training word embedding by building a sentiment classifier
- Word embedding
- One-hot encoding
- Vectorization
- N-gram representation
- Converting text into words
- Converting text into characters
- Tokenization
- Working with text data
- Deep Learning with Sequence Data and Text
- Summary
- Visualizing weights of the CNN layer
- Visualizing outputs from intermediate layers
- Understanding what a CNN model learns
- Calculating pre-convoluted features
- Training the VGG16 model
- Fine-tuning VGG16
- Freezing the layers
- Creating and exploring a VGG16 model
- Classifying dogs and cats using transfer learning
- Classifying dogs and cats – CNN from scratch
- Training the model
- Linear layer
- View
- Nonlinear activation – ReLU
- Pooling
- Conv2d
- Building a CNN model from scratch
- MNIST – getting data
- Introduction to neural networks
- Deep Learning for Computer Vision
- Summary
- Learning rate picking strategies
- Applying regularization
- Large model enough to overfit
- Baseline model
- Prepare your data
- Evaluation protocol
- Measure of success
- Problem definition and dataset creation
- Workflow of a machine learning project
- Underfitting
- Dropout
- Applying weight regularization
- Reducing the size of the network
- Getting more data
- Overfitting and underfitting
- Feature engineering
- Handling missing values
- Value normalization
- Vectorization
- Data preprocessing and feature engineering
- Data redundancy
- Time sensitivity
- Data representativeness
- K-fold validation with shuffling
- K-fold validation
- Simple holdout validation
- Training validation and test split
- Evaluating machine learning models
- Machine learning glossary
- Reinforcement learning
- Unsupervised learning
- Supervised learning
- Three kinds of machine learning problems
- Fundamentals of Machine Learning
- Summary
- Training the model
- Building the network architecture
- Loading PyTorch tensors as batches
- Loading data into PyTorch tensors
- Image classification using deep learning
- Optimizing network architecture
- Loss functions
- Model architecture for different machine learning problems
- The PyTorch way of building deep learning algorithms
- PyTorch non-linear activations
- Leaky ReLU
- ReLU
- Tanh
- Sigmoid
- Non-linear activations
- Layers – fundamental blocks of neural networks
- Deep dive into the building blocks of neural networks
- Diving Deep into Neural Networks
- Summary
- DataLoader class
- Dataset class
- Loading data
- Optimize the neural network
- Loss function
- Network implementation
- Neural network model
- Creating learnable parameters
- Creating data for our neural network
- Variables
- Tensors on GPU
- 5-D tensors
- 4-D tensors
- Slicing tensors
- 3-D tensors
- Matrix (2-D tensors)
- Vectors (1-D tensors)
- Scalar (0-D tensors)
- Data preparation
- Our first neural network
- Installing PyTorch
- Building Blocks of Neural Networks
- Summary
- PyTorch
- Deep learning frameworks
- Data and algorithms
- Hardware availability
- Why now?
- The history of deep learning
- Hype associated with deep learning
- Applications of deep learning
- Deep learning
- Examples of machine learning in real life
- Machine learning
- The history of AI
- Artificial intelligence
- Getting Started with Deep Learning Using PyTorch
- Reviews
- Get in touch
- Conventions used
- Download the color images
- Download the example code files
- To get the most out of this book
- What this book covers
- Who this book is for
- Preface
- Packt is searching for authors like you
- About the reviewer
- About the author
- Contributors
- Foreword
- PacktPub.com
- Why subscribe?
- Packt Upsell
- Dedication
- Title Page
- coverpage
- coverpage
- Title Page
- Dedication
- Packt Upsell
- Why subscribe?
- PacktPub.com
- Foreword
- Contributors
- About the author
- About the reviewer
- Packt is searching for authors like you
- Preface
- Who this book is for
- What this book covers
- To get the most out of this book
- Download the example code files
- Download the color images
- Conventions used
- Get in touch
- Reviews
- Getting Started with Deep Learning Using PyTorch
- Artificial intelligence
- The history of AI
- Machine learning
- Examples of machine learning in real life
- Deep learning
- Applications of deep learning
- Hype associated with deep learning
- The history of deep learning
- Why now?
- Hardware availability
- Data and algorithms
- Deep learning frameworks
- PyTorch
- Summary
- Building Blocks of Neural Networks
- Installing PyTorch
- Our first neural network
- Data preparation
- Scalar (0-D tensors)
- Vectors (1-D tensors)
- Matrix (2-D tensors)
- 3-D tensors
- Slicing tensors
- 4-D tensors
- 5-D tensors
- Tensors on GPU
- Variables
- Creating data for our neural network
- Creating learnable parameters
- Neural network model
- Network implementation
- Loss function
- Optimize the neural network
- Loading data
- Dataset class
- DataLoader class
- Summary
- Diving Deep into Neural Networks
- Deep dive into the building blocks of neural networks
- Layers – fundamental blocks of neural networks
- Non-linear activations
- Sigmoid
- Tanh
- ReLU
- Leaky ReLU
- PyTorch non-linear activations
- The PyTorch way of building deep learning algorithms
- Model architecture for different machine learning problems
- Loss functions
- Optimizing network architecture
- Image classification using deep learning
- Loading data into PyTorch tensors
- Loading PyTorch tensors as batches
- Building the network architecture
- Training the model
- Summary
- Fundamentals of Machine Learning
- Three kinds of machine learning problems
- Supervised learning
- Unsupervised learning
- Reinforcement learning
- Machine learning glossary
- Evaluating machine learning models
- Training validation and test split
- Simple holdout validation
- K-fold validation
- K-fold validation with shuffling
- Data representativeness
- Time sensitivity
- Data redundancy
- Data preprocessing and feature engineering
- Vectorization
- Value normalization
- Handling missing values
- Feature engineering
- Overfitting and underfitting
- Getting more data
- Reducing the size of the network
- Applying weight regularization
- Dropout
- Underfitting
- Workflow of a machine learning project
- Problem definition and dataset creation
- Measure of success
- Evaluation protocol
- Prepare your data
- Baseline model
- Large model enough to overfit
- Applying regularization
- Learning rate picking strategies
- Summary
- Deep Learning for Computer Vision
- Introduction to neural networks
- MNIST – getting data
- Building a CNN model from scratch
- Conv2d
- Pooling
- Nonlinear activation – ReLU
- View
- Linear layer
- Training the model
- Classifying dogs and cats – CNN from scratch
- Classifying dogs and cats using transfer learning
- Creating and exploring a VGG16 model
- Freezing the layers
- Fine-tuning VGG16
- Training the VGG16 model
- Calculating pre-convoluted features
- Understanding what a CNN model learns
- Visualizing outputs from intermediate layers
- Visualizing weights of the CNN layer
- Summary
- Deep Learning with Sequence Data and Text
- Working with text data
- Tokenization
- Converting text into characters
- Converting text into words
- N-gram representation
- Vectorization
- One-hot encoding
- Word embedding
- Training word embedding by building a sentiment classifier
- Downloading IMDB data and performing text tokenization
- torchtext.data
- torchtext.datasets
- Building vocabulary
- Generate batches of vectors
- Creating a network model with embedding
- Training the model
- Using pretrained word embeddings
- Downloading the embeddings
- Loading the embeddings in the model
- Freeze the embedding layer weights
- Recursive neural networks
- Understanding how RNN works with an example
- LSTM
- Long-term dependency
- LSTM networks
- Preparing the data
- Creating batches
- Creating the network
- Training the model
- Convolutional network on sequence data
- Understanding one-dimensional convolution for sequence data
- Creating the network
- Training the model
- Summary
- Generative Networks
- Neural style transfer
- Loading the data
- Creating the VGG model
- Content loss
- Style loss
- Extracting the losses
- Creating loss function for each layers
- Creating the optimizer
- Training
- Generative adversarial networks
- Deep convolutional GAN
- Defining the generator network
- Transposed convolutions
- Batch normalization
- Generator
- Defining the discriminator network
- Defining loss and optimizer
- Training the discriminator
- Training the discriminator with real images
- Training the discriminator with fake images
- Training the generator network
- Training the complete network
- Inspecting the generated images
- Language modeling
- Preparing the data
- Generating the batches
- Batches
- Backpropagation through time
- Defining a model based on LSTM
- Defining the train and evaluate functions
- Training the model
- Summary
- Modern Network Architectures
- Modern network architectures
- ResNet
- Creating PyTorch datasets
- Creating loaders for training and validation
- Creating a ResNet model
- Extracting convolutional features
- Creating a custom PyTorch dataset class for the pre-convoluted features and loader
- Creating a simple linear model
- Training and validating the model
- Inception
- Creating an Inception model
- Extracting convolutional features using register_forward_hook
- Creating a new dataset for the convoluted features
- Creating a fully connected model
- Training and validating the model
- Densely connected convolutional networks – DenseNet
- DenseBlock
- DenseLayer
- Creating a DenseNet model
- Extracting DenseNet features
- Creating a dataset and loaders
- Creating a fully connected model and train
- Model ensembling
- Creating models
- Extracting the image features
- Creating a custom dataset along with data loaders
- Creating an ensembling model
- Training and validating the model
- Encoder-decoder architecture
- Encoder
- Decoder
- Summary
- What Next?
- What next?
- Overview
- Interesting ideas to explore
- Object detection
- Image segmentation
- OpenNMT in PyTorch
- Alien NLP
- fast.ai – making neural nets uncool again
- Open Neural Network Exchange
- How to keep yourself updated
- Summary
- Other Books You May Enjoy
- Leave a review - let other readers know what you think 更新時間:2021-06-24 19:17:02