舉報
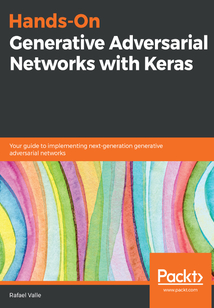
會員
Hands-On Generative Adversarial Networks with Keras
最新章節(jié):
Further reading
GenerativeAdversarialNetworks(GANs)haverevolutionizedthefieldsofmachinelearninganddeeplearning.ThisbookwillbeyourfirststeptowardsunderstandingGANarchitecturesandtacklingthechallengesinvolvedintrainingthem.Thisbookopenswithanintroductiontodeeplearningandgenerativemodels,andtheirapplicationsinartificialintelligence(AI).Youwillthenlearnhowtobuild,evaluate,andimproveyourfirstGANwiththehelpofeasy-to-followexamples.ThenextfewchapterswillguideyouthroughtrainingaGANmodeltoproduceandimprovehigh-resolutionimages.YouwillalsolearnhowtoimplementconditionalGANsthatgiveyoutheabilitytocontrolcharacteristicsofGANoutputs.YouwillbuildonyourknowledgefurtherbyexploringanewtrainingmethodologyforprogressivegrowingofGANs.Movingon,you'llgaininsightsintostate-of-the-artmodelsinimagesynthesis,speechenhancement,andnaturallanguagegenerationusingGANs.Inadditiontothis,you'llbeabletoidentifyGANsampleswithTequilaGAN.Bytheendofthisbook,youwillbewell-versedwiththelatestadvancementsintheGANframeworkusingvariousexamplesanddatasets,andyouwillhavetheskillsyouneedtoimplementGANarchitecturesforseveraltasksanddomains,includingcomputervision,naturallanguageprocessing(NLP),andaudioprocessing.ForewordbyTing-ChunWang,SeniorResearchScientist,NVIDIA
目錄(216章)
倒序
- coverpage
- Title Page
- Copyright and Credits
- Hands-On Generative Adversarial Networks with Keras
- About Packt
- Why subscribe?
- Packt.com
- Foreword
- Contributors
- About the author
- About the reviewer
- Packt is searching for authors like you
- Preface
- Who this book is for
- What this book covers
- To get the most out of this book
- Download the example code files
- Download the color images
- Conventions used
- Get in touch
- Reviews
- Section 1: Introduction and Environment Setup
- Deep Learning Basics and Environment Setup
- Deep learning basics
- Artificial Neural Networks (ANNs)
- The parameter estimation
- Backpropagation
- Loss functions
- L1 loss
- L2 loss
- Categorical crossentropy loss
- Non-linearities
- Sigmoid
- Tanh
- ReLU
- A fully connected layer
- The convolution layer
- The max pooling layer
- Deep learning environment setup
- Installing Anaconda and Python
- Setting up a virtual environment in Anaconda
- Installing TensorFlow
- Installing Keras
- Installing data visualization and machine learning libraries
- The matplotlib library
- The Jupyter library
- The scikit-learn library
- NVIDIA's CUDA Toolkit and cuDNN
- The deep learning environment test
- Summary
- Introduction to Generative Models
- Discriminative and generative models compared
- Comparing discriminative and generative models
- Generative models
- Autoregressive models
- Variational autoencoders
- Reversible flows
- Generative adversarial networks
- GANs – building blocks
- The discriminator
- The generator
- Real and fake data
- Random noise
- Discriminator and generator loss
- GANs – strengths and weaknesses
- Summary
- Section 2: Training GANs
- Implementing Your First GAN
- Technical requirements
- Imports
- Implementing a Generator and Discriminator
- Generator
- Discriminator
- Auxiliary functions
- Training your GAN
- Summary
- Further reading
- Evaluating Your First GAN
- The evaluation of GANs
- Image quality
- Image variety
- Domain specifications
- Qualitative methods
- k-nearest neighbors
- Mode analysis
- Other methods
- Quantitative methods
- The Inception score
- The Frechét Inception Distance
- Precision Recall and the F1 Score
- GANs and the birthday paradox
- Summary
- Improving Your First GAN
- Technical requirements
- Challenges in training GANs
- Mode collapse and mode drop
- Training instability
- Sensitivity to hyperparameter initialization
- Vanishing gradients
- Tricks of the trade
- Tracking failure
- Working with labels
- Working with discrete inputs
- Adding noise
- Input normalization
- Modified objective function
- Distribute latent vector
- Weight normalization
- Avoid sparse gradients
- Use a different optimizer
- Learning rate schedule
- GAN model architectures
- ResNet GAN
- GAN algorithms and loss functions
- Least Squares GAN
- Wasserstein GAN
- Wasserstein GAN with gradient penalty
- Relativistic GAN
- Summary
- Section 3: Application of GANs in Computer Vision Natural Language Processing and Audio
- Progressive Growing of GANs
- Technical requirements
- Progressive Growing of GANs
- Increasing variation using minibatch standard deviation
- Normalization in the generator and the discriminator
- Pixelwise feature vector normalization in the generator
- Experimental setup
- Training
- Helper functions
- Initializations
- Training loops
- Model implementation
- Custom layers
- The discriminator
- The generator
- GANs
- Summary
- Generation of Discrete Sequences Using GANs
- Technical requirements
- Natural language generation with GANs
- Experimental setup
- Data
- Auxiliary training functions
- Training
- Imports and global variables
- Initializations
- Training loop
- Logging
- Model implementation
- Helper functions
- Discriminator
- Generator
- Inference
- Model trained on words
- Model trained on characters
- Summary
- Text-to-Image Synthesis with GANs
- Technical Requirements
- Text-to-image synthesis
- Experimental setup
- Data utils
- Logging utils
- Training
- Initial setup
- The training loop
- Model implementation
- Wrapper
- Discriminator
- Generator
- Improving the baseline model
- Training
- Inference
- Sampling the generator
- Interpolation in the Latent Space
- Interpolation in the text-embedding space
- Inferencing with arithmetic in the text-embedding space
- Summary
- TequilaGAN - Identifying GAN Samples
- Technical requirements
- Identifying GAN samples
- Related work
- Feature extraction
- Centroid
- Slope
- Metrics
- Jensen-Shannon divergence
- Kolgomorov-Smirnov Two-Sample test
- Experiments
- MNIST
- Summary
- References
- Whats next in GANs
- What we've GANed so far
- Generative models
- Architectures
- Loss functions
- Tricks of the trade
- Implementations
- Unanswered questions in GANs
- Are some losses better than others?
- Do GANs do distribution learning?
- All about that inductive bias
- How can you kill a GAN?
- Artistic GANs
- Visual arts
- GANGogh
- Image inpainting
- Vid2Vid
- GauGAN
- Sonic arts
- MuseGAN
- GANSynth
- Recent and yet-to-be-explored GAN topics
- Summary
- Closing remarks
- Further reading 更新時間:2021-06-24 14:34:30
推薦閱讀
- 基于C語言的程序設計
- OpenStack for Architects
- RPA:流程自動化引領數(shù)字勞動力革命
- 數(shù)據(jù)挖掘方法及天體光譜挖掘技術
- 計算機系統(tǒng)結(jié)構
- Moodle Course Design Best Practices
- PVCBOT機器人控制技術入門
- Extending Ansible
- 電腦上網(wǎng)輕松入門
- 手機游戲程序開發(fā)
- 奇點將至
- 基于Proteus的單片機應用技術
- 傳感器應用技術
- fastText Quick Start Guide
- 商務智能
- CAD應用程序開發(fā)詳解
- 三維動畫制作(3ds max7.0)
- VMware vSphere 6.5 Cookbook(Third Edition)
- AI成“神”之日:人工智能的終極演變
- 單片機數(shù)據(jù)通信及測控應用技術詳解
- 操作系統(tǒng)及網(wǎng)絡應用技術
- 數(shù)碼照片處理輕松入門
- Learning D3.js Mapping
- Selenium測試實踐
- 誰說菜鳥不會數(shù)據(jù)分析:工具篇
- 機器學習技術及應用
- 單片機原理及應用
- Photoshop CS3中文版圖像處理與創(chuàng)意設計
- Practical DevOps
- Cisco ACI Cookbook