舉報
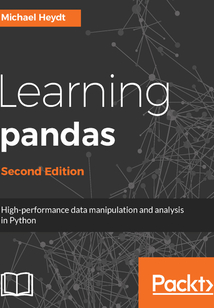
會員
Learning pandas(Second Edition)
最新章節:
Summary
Thisbookisidealfordatascientists,dataanalysts,Pythonprogrammerswhowanttoplungeintodataanalysisusingpandas,andanyonewithacuriosityaboutanalyzingdata.Someknowledgeofstatisticsandprogrammingwillbehelpfultogetthemostoutofthisbookbutnotstrictlyrequired.Priorexposuretopandasisalsonotrequired.
目錄(319章)
倒序
- coverpage
- Title Page
- Credits
- About the Author
- About the Reviewers
- www.PacktPub.com
- Why subscribe?
- Customer Feedback
- Preface
- What this book covers
- What you need for this book
- Who this book is for
- Conventions
- Reader feedback
- Customer support
- Downloading the example code
- Errata
- Piracy
- Questions
- pandas and Data Analysis
- Introducing pandas
- Data manipulation analysis science and pandas
- Data manipulation
- Data analysis
- Data science
- Where does pandas fit?
- The process of data analysis
- The process
- Ideation
- Retrieval
- Preparation
- Exploration
- Modeling
- Presentation
- Reproduction
- A note on being iterative and agile
- Relating the book to the process
- Concepts of data and analysis in our tour of pandas
- Types of data
- Structured
- Unstructured
- Semi-structured
- Variables
- Categorical
- Continuous
- Discrete
- Time series data
- General concepts of analysis and statistics
- Quantitative versus qualitative data/analysis
- Single and multivariate analysis
- Descriptive statistics
- Inferential statistics
- Stochastic models
- Probability and Bayesian statistics
- Correlation
- Regression
- Other Python libraries of value with pandas
- Numeric and scientific computing - NumPy and SciPy
- Statistical analysis – StatsModels
- Machine learning – scikit-learn
- PyMC - stochastic Bayesian modeling
- Data visualization - matplotlib and seaborn
- Matplotlib
- Seaborn
- Summary
- Up and Running with pandas
- Installation of Anaconda
- IPython and Jupyter Notebook
- IPython
- Jupyter Notebook
- Introducing the pandas Series and DataFrame
- Importing pandas
- The pandas Series
- The pandas DataFrame
- Loading data from files into a DataFrame
- Visualization
- Summary
- Representing Univariate Data with the Series
- Configuring pandas
- Creating a Series
- Creating a Series using Python lists and dictionaries
- Creation using NumPy functions
- Creation using a scalar value
- The .index and .values properties
- The size and shape of a Series
- Specifying an index at creation
- Heads tails and takes
- Retrieving values in a Series by label or position
- Lookup by label using the [] operator and the .ix[] property
- Explicit lookup by position with .iloc[]
- Explicit lookup by labels with .loc[]
- Slicing a Series into subsets
- Alignment via index labels
- Performing Boolean selection
- Re-indexing a Series
- Modifying a Series in-place
- Summary
- Representing Tabular and Multivariate Data with the DataFrame
- Configuring pandas
- Creating DataFrame objects
- Creating a DataFrame using NumPy function results
- Creating a DataFrame using a Python dictionary and pandas Series objects
- Creating a DataFrame from a CSV file
- Accessing data within a DataFrame
- Selecting the columns of a DataFrame
- Selecting rows of a DataFrame
- Scalar lookup by label or location using .at[] and .iat[]
- Slicing using the [ ] operator
- Selecting rows using Boolean selection
- Selecting across both rows and columns
- Summary
- Manipulating DataFrame Structure
- Configuring pandas
- Renaming columns
- Adding new columns with [] and .insert()
- Adding columns through enlargement
- Adding columns using concatenation
- Reordering columns
- Replacing the contents of a column
- Deleting columns
- Appending new rows
- Concatenating rows
- Adding and replacing rows via enlargement
- Removing rows using .drop()
- Removing rows using Boolean selection
- Removing rows using a slice
- Summary
- Indexing Data
- Configuring pandas
- The importance of indexes
- The pandas index types
- The fundamental type - Index
- Integer index labels using Int64Index and RangeIndex
- Floating-point labels using Float64Index
- Representing discrete intervals using IntervalIndex
- Categorical values as an index - CategoricalIndex
- Indexing by date and time using DatetimeIndex
- Indexing periods of time using PeriodIndex
- Working with Indexes
- Creating and using an index with a Series or DataFrame
- Selecting values using an index
- Moving data to and from the index
- Reindexing a pandas object
- Hierarchical indexing
- Summary
- Categorical Data
- Configuring pandas
- Creating Categoricals
- Renaming categories
- Appending new categories
- Removing categories
- Removing unused categories
- Setting categories
- Descriptive information of a Categorical
- Munging school grades
- Summary
- Numerical and Statistical Methods
- Configuring pandas
- Performing numerical methods on pandas objects
- Performing arithmetic on a DataFrame or Series
- Getting the counts of values
- Determining unique values (and their counts)
- Finding minimum and maximum values
- Locating the n-smallest and n-largest values
- Calculating accumulated values
- Performing statistical processes on pandas objects
- Retrieving summary descriptive statistics
- Measuring central tendency: mean median and mode
- Calculating the mean
- Finding the median
- Determining the mode
- Calculating variance and standard deviation
- Measuring variance
- Finding the standard deviation
- Determining covariance and correlation
- Calculating covariance
- Determining correlation
- Performing discretization and quantiling of data
- Calculating the rank of values
- Calculating the percent change at each sample of a series
- Performing moving-window operations
- Executing random sampling of data
- Summary
- Accessing Data
- Configuring pandas
- Working with CSV and text/tabular format data
- Examining the sample CSV data set
- Reading a CSV file into a DataFrame
- Specifying the index column when reading a CSV file
- Data type inference and specification
- Specifying column names
- Specifying specific columns to load
- Saving DataFrame to a CSV file
- Working with general field-delimited data
- Handling variants of formats in field-delimited data
- Reading and writing data in Excel format
- Reading and writing JSON files
- Reading HTML data from the web
- Reading and writing HDF5 format files
- Accessing CSV data on the web
- Reading and writing from/to SQL databases
- Reading data from remote data services
- Reading stock data from Yahoo! and Google Finance
- Retrieving options data from Google Finance
- Reading economic data from the Federal Reserve Bank of St. Louis
- Accessing Kenneth French's data
- Reading from the World Bank
- Summary
- Tidying Up Your Data
- Configuring pandas
- What is tidying your data?
- How to work with missing data
- Determining NaN values in pandas objects
- Selecting out or dropping missing data
- Handling of NaN values in mathematical operations
- Filling in missing data
- Forward and backward filling of missing values
- Filling using index labels
- Performing interpolation of missing values
- Handling duplicate data
- Transforming data
- Mapping data into different values
- Replacing values
- Applying functions to transform data
- Summary
- Combining Relating and Reshaping Data
- Configuring pandas
- Concatenating data in multiple objects
- Understanding the default semantics of concatenation
- Switching axes of alignment
- Specifying join type
- Appending versus concatenation
- Ignoring the index labels
- Merging and joining data
- Merging data from multiple pandas objects
- Specifying the join semantics of a merge operation
- Pivoting data to and from value and indexes
- Stacking and unstacking
- Stacking using non-hierarchical indexes
- Unstacking using hierarchical indexes
- Melting data to and from long and wide format
- Performance benefits of stacked data
- Summary
- Data Aggregation
- Configuring pandas
- The split apply and combine (SAC) pattern
- Data for the examples
- Splitting data
- Grouping by a single column's values
- Accessing the results of a grouping
- Grouping using multiple columns
- Grouping using index levels
- Applying aggregate functions transforms and filters
- Applying aggregation functions to groups
- Transforming groups of data
- The general process of transformation
- Filling missing values with the mean of the group
- Calculating normalized z-scores with a transformation
- Filtering groups from aggregation
- Summary
- Time-Series Modelling
- Setting up the IPython notebook
- Representation of dates time and intervals
- The datetime day and time objects
- Representing a point in time with a Timestamp
- Using a Timedelta to represent a time interval
- Introducing time-series data
- Indexing using DatetimeIndex
- Creating time-series with specific frequencies
- Calculating new dates using offsets
- Representing data intervals with date offsets
- Anchored offsets
- Representing durations of time using Period
- Modelling an interval of time with a Period
- Indexing using the PeriodIndex
- Handling holidays using calendars
- Normalizing timestamps using time zones
- Manipulating time-series data
- Shifting and lagging
- Performing frequency conversion on a time-series
- Up and down resampling of a time-series
- Time-series moving-window operations
- Summary
- Visualization
- Configuring pandas
- Plotting basics with pandas
- Creating time-series charts
- Adorning and styling your time-series plot
- Adding a title and changing axes labels
- Specifying the legend content and position
- Specifying line colors styles thickness and markers
- Specifying tick mark locations and tick labels
- Formatting axes' tick date labels using formatters
- Common plots used in statistical analyses
- Showing relative differences with bar plots
- Picturing distributions of data with histograms
- Depicting distributions of categorical data with box and whisker charts
- Demonstrating cumulative totals with area plots
- Relationships between two variables with scatter plots
- Estimates of distribution with the kernel density plot
- Correlations between multiple variables with the scatter plot matrix
- Strengths of relationships in multiple variables with heatmaps
- Manually rendering multiple plots in a single chart
- Summary
- Historical Stock Price Analysis
- Setting up the IPython notebook
- Obtaining and organizing stock data from Google
- Plotting time-series prices
- Plotting volume-series data
- Calculating the simple daily percentage change in closing price
- Calculating simple daily cumulative returns of a stock
- Resampling data from daily to monthly returns
- Analyzing distribution of returns
- Performing a moving-average calculation
- Comparison of average daily returns across stocks
- Correlation of stocks based on the daily percentage change of the closing price
- Calculating the volatility of stocks
- Determining risk relative to expected returns
- Summary 更新時間:2021-07-02 20:38:38
推薦閱讀
- 無代碼編程:用云表搭建企業數字化管理平臺
- Mastering Kotlin
- Mastering Julia
- Blockly創意趣味編程
- The Computer Vision Workshop
- Visual Basic程序設計實驗指導(第4版)
- Java實戰(第2版)
- 劍指大數據:企業級數據倉庫項目實戰(在線教育版)
- Internet of Things with ESP8266
- Unity&VR游戲美術設計實戰
- 微課學人工智能Python編程
- Orchestrating Docker
- Visual Basic程序設計全程指南
- IBM RUP參考與認證指南
- FusionCharts Beginner’s Guide:The Official Guide for FusionCharts Suite
- Head First Kotlin程序設計
- ANSYS FLUENT 16.0超級學習手冊
- Practical Responsive Typography
- Instant SQL Server Analysis Services 2012 Cube Security
- Neo4j High Performance
- Nginx Troubleshooting
- Go語言從入門到項目實戰(視頻版)
- 寫給UI設計師看的數據可視化設計
- C語言項目式系統開發教程(微課版)
- C#程序設計教程
- Piwik Web Analytics Essentials
- 零基礎看圖學Scratch 3.0少兒趣味編程(全彩大字版)
- GameMaker Cookbook
- 編程超有趣:奇妙Python輕松學(第1輯)
- AngularJS Directives