舉報
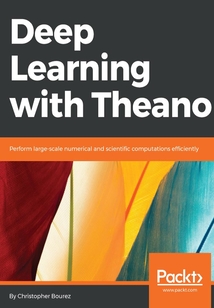
會員
Deep Learning with Theano
最新章節(jié):
Index
Thisbookisindentedtoprovideafulloverviewofdeeplearning.Fromthebeginnerindeeplearningandartificialintelligence,tothedatascientistwhowantstobecomefamiliarwithTheanoanditssupportinglibraries,orhaveanextendedunderstandingofdeepneuralnets.SomebasicskillsinPythonprogrammingandcomputersciencewillhelp,aswellasskillsinelementaryalgebraandcalculus.
目錄(139章)
倒序
- 封面
- 書名頁
- Deep Learning with Theano
- Credits
- About the Author
- Acknowledgments
- About the Reviewers
- www.PacktPub.com
- eBooks discount offers and more
- Customer Feedback
- Preface
- What this book covers
- What you need for this book
- Who this book is for
- Conventions
- Reader feedback
- Customer support
- Chapter 1. Theano Basics
- The need for tensors
- Installing and loading Theano
- Tensors
- Graphs and symbolic computing
- Operations on tensors
- Memory and variables
- Functions and automatic differentiation
- Loops in symbolic computing
- Configuration profiling and debugging
- Summary
- Chapter 2. Classifying Handwritten Digits with a Feedforward Network
- The MNIST dataset
- Structure of a training program
- Classification loss function
- Single-layer linear model
- Cost function and errors
- Backpropagation and stochastic gradient descent
- Multiple layer model
- Convolutions and max layers
- Training
- Dropout
- Inference
- Optimization and other update rules
- Related articles
- Summary
- Chapter 3. Encoding Word into Vector
- Encoding and embedding
- Dataset
- Continuous Bag of Words model
- Training the model
- Visualizing the learned embeddings
- Evaluating embeddings – analogical reasoning
- Evaluating embeddings – quantitative analysis
- Application of word embeddings
- Weight tying
- Further reading
- Summary
- Chapter 4. Generating Text with a Recurrent Neural Net
- Need for RNN
- A dataset for natural language
- Simple recurrent network
- Metrics for natural language performance
- Training loss comparison
- Example of predictions
- Applications of RNN
- Related articles
- Summary
- Chapter 5. Analyzing Sentiment with a Bidirectional LSTM
- Installing and configuring Keras
- Preprocessing text data
- Designing the architecture for the model
- Compiling and training the model
- Evaluating the model
- Saving and loading the model
- Running the example
- Further reading
- Summary
- Chapter 6. Locating with Spatial Transformer Networks
- MNIST CNN model with Lasagne
- A localization network
- Unsupervised learning with co-localization
- Region-based localization networks
- Further reading
- Summary
- Chapter 7. Classifying Images with Residual Networks
- Natural image datasets
- Residual connections
- Stochastic depth
- Dense connections
- Multi-GPU
- Data augmentation
- Further reading
- Summary
- Chapter 8. Translating and Explaining with Encoding – decoding Networks
- Sequence-to-sequence networks for natural language processing
- Seq2seq for translation
- Seq2seq for chatbots
- Improving efficiency of sequence-to-sequence network
- Deconvolutions for images
- Multimodal deep learning
- Further reading
- Summary
- Chapter 9. Selecting Relevant Inputs or Memories with the Mechanism of Attention
- Differentiable mechanism of attention
- Store and retrieve information in Neural Turing Machines
- Memory networks
- Further reading
- Summary
- Chapter 10. Predicting Times Sequences with Advanced RNN
- Dropout for RNN
- Deep approaches for RNN
- Stacked recurrent networks
- Deep transition recurrent network
- Highway networks design principle
- Recurrent Highway Networks
- Further reading
- Summary
- Chapter 11. Learning from the Environment with Reinforcement
- Reinforcement learning tasks
- Simulation environments
- Q-learning
- Deep Q-network
- Training stability
- Policy gradients with REINFORCE algorithms
- Related articles
- Summary
- Chapter 12. Learning Features with Unsupervised Generative Networks
- Generative models
- Semi-supervised learning
- Further reading
- Summary
- Chapter 13. Extending Deep Learning with Theano
- Theano Op in Python for CPU
- Theano Op in Python for the GPU
- Theano Op in C for CPU
- Theano Op in C for GPU
- Coalesced transpose via shared memory NVIDIA parallel for all
- The future of artificial intelligence
- Further reading
- Summary
- Index 更新時間:2021-07-15 17:17:25
推薦閱讀
- Python機器學習:數(shù)據(jù)分析與評分卡建模(微課版)
- vSphere High Performance Cookbook
- Julia機器學習核心編程:人人可用的高性能科學計算
- Python機器學習實戰(zhàn)
- 精通Linux(第2版)
- Yii Project Blueprints
- 基于ARM Cortex-M4F內(nèi)核的MSP432 MCU開發(fā)實踐
- C#開發(fā)案例精粹
- Microsoft 365 Certified Fundamentals MS-900 Exam Guide
- 寫給大家看的Midjourney設(shè)計書
- 產(chǎn)品架構(gòu)評估原理與方法
- 軟件再工程:優(yōu)化現(xiàn)有軟件系統(tǒng)的方法與最佳實踐
- C#程序開發(fā)參考手冊
- C++ Data Structures and Algorithm Design Principles
- Django 2.0 入門與實踐
- 數(shù)據(jù)庫基礎(chǔ)與應用實驗教程:Visual FoxPro 6.0
- Microsoft Hyper-V PowerShell Automation
- Python游戲編程項目開發(fā)實戰(zhàn)
- 機器學習開發(fā)者指南
- Mastering WooCommerce 4
- 性能之道:分布式系統(tǒng)全棧性能優(yōu)化
- 新印象:中文版Sketch圖標與UI界面設(shè)計實例教程
- 游戲概論
- 大學信息基礎(chǔ)
- IBM Informix 11.x系統(tǒng)管理與開發(fā)指南
- JavaScript深度學習
- Access 2010數(shù)據(jù)庫項目應用教程
- WCF Multi-layer Services Development with Entity Framework(Fourth Edition)
- Automating Microsoft Azure with PowerShell
- 自動化運維軟件設(shè)計實戰(zhàn)