舉報(bào)
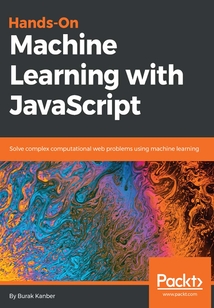
會員
Hands-On Machine Learning with JavaScript
ThisbookisforyouifyouareaJavaScriptdeveloperwhowantstoimplementmachinelearningtomakeapplicationssmarter,gaininsightfulinformationfromthedata,andenterthefieldofmachinelearningwithoutswitchingtoanotherlanguage.WorkingknowledgeofJavaScriptlanguageisexpectedtogetthemostoutofthebook.
目錄(157章)
倒序
- 封面
- 版權(quán)信息
- Packt Upsell
- Why subscribe?
- PacktPub.com
- Contributors
- About the author
- About the reviewer
- Packt is searching for authors like you
- Preface
- Who this book is for
- What this book covers
- To get the most out of this book
- Download the example code files
- Download the color images
- Conventions used
- Get in touch
- Reviews
- Exploring the Potential of JavaScript
- Why JavaScript?
- Why machine learning why now?
- Advantages and challenges of JavaScript
- The CommonJS initiative
- Node.js
- TypeScript language
- Improvements in ES6
- Let and const
- Classes
- Module imports
- Arrow functions
- Object literals
- The for...of function
- Promises
- The async/await functions
- Preparing the development environment
- Installing Node.js
- Optionally installing Yarn
- Creating and initializing an example project
- Creating a Hello World project
- Summary
- Data Exploration
- An overview
- Feature identification
- The curse of dimensionality
- Feature selection and feature extraction
- Pearson correlation example
- Cleaning and preparing data
- Handling missing data
- Missing categorical data
- Missing numerical data
- Handling noise
- Handling outliers
- Transforming and normalizing data
- Summary
- Tour of Machine Learning Algorithms
- Introduction to machine learning
- Types of learning
- Unsupervised learning
- Supervised learning
- Measuring accuracy
- Supervised learning algorithms
- Reinforcement learning
- Categories of algorithms
- Clustering
- Classification
- Regression
- Dimensionality reduction
- Optimization
- Natural language processing
- Image processing
- Summary
- Grouping with Clustering Algorithms
- Average and distance
- Writing the k-means algorithm
- Setting up the environment
- Initializing the algorithm
- Testing random centroid generation
- Assigning points to centroids
- Updating centroid locations
- The main loop
- Example 1 – k-means on simple 2D data
- Example 2 – 3D data
- k-means where k is unknown
- Summary
- Classification Algorithms
- k-Nearest Neighbor
- Building the KNN algorithm
- Example 1 – Height weight and gender
- Example 2 – Decolorizing a photo
- Naive Bayes classifier
- Tokenization
- Building the algorithm
- Example 3 – Movie review sentiment
- Support Vector Machine
- Random forest
- Summary
- Association Rule Algorithms
- The mathematical perspective
- The algorithmic perspective
- Association rule applications
- Example – retail data
- Summary
- Forecasting with Regression Algorithms
- Regression versus classification
- Regression basics
- Example 1 – linear regression
- Example 2 – exponential regression
- Example 3 – polynomial regression
- Other time-series analysis techniques
- Filtering
- Seasonality analysis
- Fourier analysis
- Summary
- Artificial Neural Network Algorithms
- Conceptual overview of neural networks
- Backpropagation training
- Example - XOR in TensorFlow.js
- Summary
- Deep Neural Networks
- Convolutional Neural Networks
- Convolutions and convolution layers
- Example – MNIST handwritten digits
- Recurrent neural networks
- SimpleRNN
- Gated recurrent units
- Long Short-Term Memory
- Summary
- Natural Language Processing in Practice
- String distance
- Term frequency - inverse document frequency
- Tokenizing
- Stemming
- Phonetics
- Part of speech tagging
- Word embedding and neural networks
- Summary
- Using Machine Learning in Real-Time Applications
- Serializing models
- Training models on the server
- Web workers
- Continually improving and per-user models
- Data pipelines
- Data querying
- Data joining and aggregation
- Transformation and normalization
- Storing and delivering data
- Summary
- Choosing the Best Algorithm for Your Application
- Mode of learning
- The task at hand
- Format form input and output
- Available resources
- When it goes wrong
- Combining models
- Summary
- Other Books You May Enjoy
- Leave a review - let other readers know what you think 更新時(shí)間:2021-06-25 21:38:42
推薦閱讀
- Dreamweaver CS3+Flash CS3+Fireworks CS3創(chuàng)意網(wǎng)站構(gòu)建實(shí)例詳解
- Mastering Hadoop 3
- 人工智能超越人類
- 會聲會影X5視頻剪輯高手速成
- 機(jī)器自動化控制器原理與應(yīng)用
- 新手學(xué)電腦快速入門
- Statistics for Data Science
- 網(wǎng)絡(luò)安全技術(shù)及應(yīng)用
- Mastering Ceph
- 計(jì)算智能算法及其生產(chǎn)調(diào)度應(yīng)用
- 菜鳥起飛電腦組裝·維護(hù)與故障排查
- 網(wǎng)管員世界2009超值精華本
- 特征工程入門與實(shí)踐
- ASP.NET學(xué)習(xí)手冊
- 工業(yè)控制系統(tǒng)安全
- 數(shù)據(jù)庫技術(shù)及應(yīng)用
- 實(shí)戰(zhàn)Hadoop
- Geospatial Data Science Quick Start Guide
- IBM Watson Projects
- 黑客攻防實(shí)戰(zhàn)編程
- 仿人機(jī)器人開發(fā)指南
- 微機(jī)原理與接口技術(shù)
- 電氣控制及PLC技術(shù):羅克韋爾Micro800系列
- Hands-On Neural Networks
- Ubuntu 20.04 Essentials
- Visual C++.NET串口通信及測控應(yīng)用典型實(shí)例
- Mastering PostgreSQL 12
- CentOS System Administration Essentials
- 深入淺出PyTorch:從模型到源碼
- Hands-On Recommendation Systems with Python