舉報
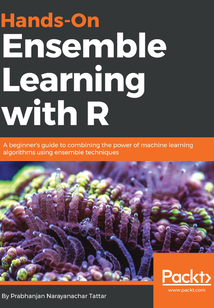
會員
Hands-On Ensemble Learning with R
最新章節:
R package references
Ensembletechniquesareusedforcombiningtwoormoresimilarordissimilarmachinelearningalgorithmstocreateastrongermodel.Suchamodeldeliverssuperiorpredictionpowerandcangiveyourdatasetsaboostinaccuracy.Hands-OnEnsembleLearningwithRbeginswiththeimportantstatisticalresamplingmethods.Youwillthenwalkthroughthecentraltrilogyofensembletechniques–bagging,randomforest,andboosting–thenyou'lllearnhowtheycanbeusedtoprovidegreateraccuracyonlargedatasetsusingpopularRpackages.Youwilllearnhowtocombinemodelpredictionsusingdifferentmachinelearningalgorithmstobuildensemblemodels.Inadditiontothis,youwillexplorehowtoimprovetheperformanceofyourensemblemodels.Bytheendofthisbook,youwillhavelearnedhowmachinelearningalgorithmscanbecombinedtoreducecommonproblemsandbuildsimpleefficientensemblemodelswiththehelpofreal-worldexamples.
目錄(155章)
倒序
- 封面
- Hands-On Ensemble Learning with R
- Why subscribe?
- PacktPub.com
- Contributors
- About the author
- About the reviewer
- Packt is Searching for Authors Like You
- Preface
- Who this book is for
- What this book covers
- To get the most out of this book
- Download the example code files
- Download the color images
- Conventions used
- Get in touch
- Reviews
- Chapter 1. Introduction to Ensemble Techniques
- Datasets
- Hypothyroid
- Waveform
- German Credit
- Iris
- Pima Indians Diabetes
- US Crime
- Overseas visitors
- Primary Biliary Cirrhosis
- Multishapes
- Board Stiffness
- Statistical/machine learning models
- Logistic regression model
- Neural networks
- Na?ve Bayes classifier
- Decision tree
- Support vector machines
- The right model dilemma!
- An ensemble purview
- Complementary statistical tests
- Permutation test
- Chi-square and McNemar test
- ROC test
- Summary
- Chapter 2. Bootstrapping
- Technical requirements
- The jackknife technique
- The jackknife method for mean and variance
- Pseudovalues method for survival data
- Bootstrap – a statistical method
- The standard error of correlation coefficient
- The parametric bootstrap
- Eigen values
- The boot package
- Bootstrap and testing hypotheses
- Bootstrapping regression models
- Bootstrapping survival models*
- Bootstrapping time series models*
- Summary
- Chapter 3. Bagging
- Technical requirements
- Classification trees and pruning
- Bagging
- k-NN classifier
- Analyzing waveform data
- k-NN bagging
- Summary
- Chapter 4. Random Forests
- Technical requirements
- Random Forests
- Variable importance
- Proximity plots
- Random Forest nuances
- Comparisons with bagging
- Missing data imputation
- Clustering with Random Forest
- Summary
- Chapter 5. The Bare Bones Boosting Algorithms
- Technical requirements
- The general boosting algorithm
- Adaptive boosting
- Gradient boosting
- Using the adabag and gbm packages
- Variable importance
- Comparing bagging random forests and boosting
- Summary
- Chapter 6. Boosting Refinements
- Technical requirements
- Why does boosting work?
- The gbm package
- Boosting for count data
- Boosting for survival data
- The xgboost package
- The h1o package
- Summary
- Chapter 7. The General Ensemble Technique
- Technical requirements
- Why does ensembling work?
- Ensembling by voting
- Majority voting
- Weighted voting
- Ensembling by averaging
- Simple averaging
- Weight averaging
- Stack ensembling
- Summary
- Chapter 8. Ensemble Diagnostics
- Technical requirements
- What is ensemble diagnostics?
- Ensemble diversity
- Numeric prediction
- Pairwise measure
- Disagreement measure
- Yule's or Q-statistic
- Correlation coefficient measure
- Cohen's statistic
- Double-fault measure
- Interrating agreement
- Entropy measure
- Kohavi-Wolpert measure
- Disagreement measure for ensemble
- Measurement of interrater agreement
- Summary
- Chapter 9. Ensembling Regression Models
- Technical requirements
- Pre-processing the housing data
- Visualization and variable reduction
- Variable clustering
- Regression models
- Linear regression model
- Neural networks
- Regression tree
- Prediction for regression models
- Bagging and Random Forests
- Boosting regression models
- Stacking methods for regression models
- Summary
- Chapter 10. Ensembling Survival Models
- Core concepts of survival analysis
- Nonparametric inference
- Regression models – parametric and Cox proportional hazards models
- Survival tree
- Ensemble survival models
- Summary
- Chapter 11. Ensembling Time Series Models
- Technical requirements
- Time series datasets
- Time series visualization
- Core concepts and metrics
- Essential time series models
- Bagging and time series
- Ensemble time series models
- Summary
- Chapter 12. What's Next?
- Appendix A. Bibliography
- References
- R package references 更新時間:2021-07-23 19:11:24
推薦閱讀
- Microsoft Power BI Quick Start Guide
- 會聲會影X5視頻剪輯高手速成
- 人工免疫算法改進及其應用
- Photoshop CS3圖像處理融會貫通
- 大型數據庫管理系統技術、應用與實例分析:SQL Server 2005
- 精通特征工程
- 人工智能實踐錄
- Extending Ansible
- Learning Linux Shell Scripting
- 中國戰略性新興產業研究與發展·數控系統
- WPF專業編程指南
- 網管員世界2009超值精華本
- Win 7二十一
- Office 2010輕松入門
- 單片機硬件接口電路及實例解析
- 多傳感器數據智能融合理論與應用
- Mastering Windows Group Policy
- Proteus從入門到精通100例
- 小數據之美:精準捕捉未來的商業小趨勢
- S7-200系列PLC應用技術
- 數字媒體交互設計原理與方法
- 工業機器人實戰應用及調試
- Learning QGIS(Third Edition)
- Drupal 7 Multi Sites Configuration
- 零基礎學三菱PLC編程:入門、提高、應用、實例
- Hands-On Predictive Analytics with Python
- PHP+MySQL+AJAX Web開發給力起飛
- Practical Site Reliability Engineering
- 數據庫應用基礎:Access 2007
- 自己動手做交互系統