舉報
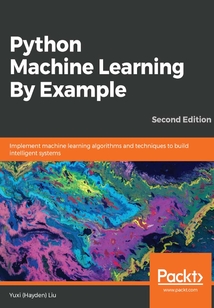
會員
Python Machine Learning By Example
Thesurgeininterestinmachinelearning(ML)isduetothefactthatitrevolutionizesautomationbylearningpatternsindataandusingthemtomakepredictionsanddecisions.Ifyou’reinterestedinML,thisbookwillserveasyourentrypointtoML.PythonMachineLearningByExamplebeginswithanintroductiontoimportantMLconceptsandimplementationsusingPythonlibraries.Eachchapterofthebookwalksyouthroughanindustryadoptedapplication.You’llimplementMLtechniquesinareassuchasexploratorydataanalysis,featureengineering,andnaturallanguageprocessing(NLP)inaclearandeasy-to-followway.Withthehelpofthisextendedandupdatededition,you’llunderstandhowtotackledata-drivenproblemsandimplementyoursolutionswiththepowerfulyetsimplePythonlanguageandpopularPythonpackagesandtoolssuchasTensorFlow,scikit-learn,gensim,andKeras.ToaidyourunderstandingofpopularMLalgorithms,thebookcoversinterestingandeasy-to-followexamplessuchasnewstopicmodelingandclassification,spamemaildetection,stockpriceforecasting,andmore.Bytheendofthebook,you’llhaveputtogetherabroadpictureoftheMLecosystemandwillbewell-versedwiththebestpracticesofapplyingMLtechniquestomakethemostoutofnewopportunities.
最新章節(jié)
- Leave a review - let other readers know what you think
- Other Books You May Enjoy
- Exercises
- Summary
- Best practice 21 – updating models regularly
- Best practice 20 – monitoring model performance
品牌:中圖公司
上架時間:2021-07-02 12:23:11
出版社:Packt Publishing
本書數(shù)字版權(quán)由中圖公司提供,并由其授權(quán)上海閱文信息技術(shù)有限公司制作發(fā)行
- Leave a review - let other readers know what you think 更新時間:2021-07-02 12:42:13
- Other Books You May Enjoy
- Exercises
- Summary
- Best practice 21 – updating models regularly
- Best practice 20 – monitoring model performance
- Best practice 19 – saving loading and reusing models
- Best practices in the deployment and monitoring stage
- Best practice 18 – modeling on large-scale datasets
- Best practice 17 – diagnosing overfitting and underfitting
- Best practice 16 – reducing overfitting
- Neural networks
- Random forest (or decision tree)
- SVM
- Logistic regression
- Na?ve Bayes
- Best practice 15 – choosing the right algorithm(s) to start with
- Best practices in the model training evaluation and selection stage
- Best practice 14 – extracting features from text data
- Best practice 13 – documenting how each feature is generated
- Best practice 12 – performing feature engineering without domain expertise
- Best practice 11 – performing feature engineering with domain expertise
- Best practice 10 – deciding on whether or not to rescale features
- Best practice 9 – deciding on whether or not to reduce dimensionality and if so how to do so
- Best practice 8 – deciding on whether or not to select features and if so how to do so
- Best practice 7 – deciding on whether or not to encode categorical features
- Best practice 6 – identifying categorical features with numerical values
- Best practices in the training sets generation stage
- Best practice 5 – storing large-scale data
- Best practice 4 – dealing with missing data
- Best practice 3 – maintaining the consistency of field values
- Best practice 2 – collecting all fields that are relevant
- Best practice 1 – completely understanding the project goal
- Best practices in the data preparation stage
- Machine learning solution workflow
- Machine Learning Best Practices
- Section 3: Python Machine Learning Best Practices
- Exercise
- Summary
- Predicting stock price with four regression algorithms
- Evaluating regression performance
- Implementing neural networks
- Demystifying neural networks
- Estimating with neural networks
- Implementing SVR
- Estimating with support vector regression
- Implementing regression forest
- Implementing decision tree regression
- Transitioning from classification trees to regression trees
- Estimating with decision tree regression
- Implementing linear regression
- How does linear regression work?
- Estimating with linear regression
- Acquiring data and generating features
- Getting started with feature engineering
- Mining stock price data
- What is regression?
- Brief overview of the stock market and stock prices
- Stock Price Prediction with Regression Algorithms
- Exercises
- Summary
- Combining multiple variables – feature interaction
- Hashing categorical features
- Feature engineering on categorical variables with Spark
- Training and testing a logistic regression model
- One-hot encoding categorical features
- Splitting and caching the data
- Loading click logs
- Learning on massive click logs with Spark
- Programming in PySpark
- Launching and deploying Spark programs
- Installing Spark
- Breaking down Spark
- Learning the essentials of Apache Spark
- Scaling Up Prediction to Terabyte Click Logs
- Exercises
- Summary
- Feature selection using random forest
- Implementing logistic regression using TensorFlow
- Handling multiclass classification
- Training on large datasets with online learning
- Training a logistic regression model with regularization
- Training a logistic regression model using stochastic gradient descent
- Predicting ad click-through with logistic regression using gradient descent
- Training a logistic regression model using gradient descent
- Training a logistic regression model
- Jumping from the logistic function to logistic regression
- Getting started with the logistic function
- Classifying data with logistic regression
- Converting categorical features to numerical – one-hot encoding and ordinal encoding
- Predicting Online Ad Click-Through with Logistic Regression
- Exercise
- Summary
- Implementing random forest using TensorFlow
- Ensembling decision trees – random forest
- Predicting ad click-through with decision tree
- Implementing a decision tree from scratch
- The metrics for measuring a split
- Constructing a decision tree
- Exploring decision tree from root to leaves
- Getting started with two types of data – numerical and categorical
- Brief overview of advertising click-through prediction
- Predicting Online Ad Click-Through with Tree-Based Algorithms
- Exercise
- Summary
- A further example – breast cancer classification using SVM with TensorFlow
- More example – fetal state classification on cardiotocography
- Classifying newsgroup topics with SVMs
- Choosing between linear and RBF kernels
- Case 5 – solving linearly non-separable problems
- The kernels of SVM
- Case 4 – dealing with more than two classes
- Implementing SVM
- Case 3 – handling outliers
- Case 2 – determining the optimal hyperplane
- Case 1 – identifying a separating hyperplane
- Understanding how SVM works through different use cases
- Finding separating boundary with support vector machines
- Classifying Newsgroup Topics with Support Vector Machines
- Exercise
- Summary
- Model tuning and cross-validation
- Classification performance evaluation
- Implementing Na?ve Bayes with scikit-learn
- Implementing Na?ve Bayes from scratch
- The mechanics of Na?ve Bayes
- Learning Bayes' theorem by examples
- Exploring Na?ve Bayes
- Applications of text classification
- Types of classification
- Getting started with classification
- Detecting Spam Email with Naive Bayes
- Exercises
- Summary
- Topic modeling using LDA
- Topic modeling using NMF
- Discovering underlying topics in newsgroups
- Clustering newsgroups data using k-means
- Choosing the value of k
- Implementing k-means with scikit-learn
- Implementing k-means from scratch
- How does k-means clustering work?
- Clustering newsgroups data using k-means
- Learning without guidance – unsupervised learning
- Mining the 20 Newsgroups Dataset with Clustering and Topic Modeling Algorithms
- Exercises
- Summary
- t-SNE for dimensionality reduction
- What is dimensionality reduction?
- Visualizing the newsgroups data with t-SNE
- Stemming and lemmatizing words
- Dropping stop words
- Text preprocessing
- Counting the occurrence of each word token
- Thinking about features for text data
- Exploring the newsgroups data
- Getting the newsgroups data
- Semantics and topic modeling
- Stemming and lemmatization
- Named-entity recognition
- PoS tagging
- Tokenization
- Corpus
- Picking up NLP basics while touring popular NLP libraries
- How computers understand language - NLP
- Exploring the 20 Newsgroups Dataset with Text Analysis Techniques
- Section 2: Practical Python Machine Learning By Example
- Exercises
- Summary
- TensorFlow
- Scikit-learn
- Pandas
- SciPy
- NumPy
- Installing the various packages
- Setting up Python and environments
- Installing software and setting up
- Stacking
- Boosting
- Bagging
- Voting and averaging
- Combining models
- Binning
- Power transform
- Polynomial features
- Scaling
- One hot encoding
- Label encoding
- Missing values
- Preprocessing exploration and feature engineering
- Avoiding overfitting with feature selection and dimensionality reduction
- Avoiding overfitting with regularization
- Avoiding overfitting with cross-validation
- Overfitting underfitting and the bias-variance trade-off
- Core of machine learning – generalizing with data
- A brief history of the development of machine learning algorithms
- Types of machine learning tasks
- A very high-level overview of machine learning technology
- Defining machine learning and why we need it
- Getting Started with Machine Learning and Python
- Section 1: Fundamentals of Machine Learning
- Reviews
- Get in touch
- Conventions used
- Download the color images
- Download the example code files
- To get the most out of this book
- What this book covers
- Who this book is for
- Preface
- Packt is searching for authors like you
- About the reviewer
- About the author
- Contributors
- Foreword
- Dedication
- Packt.com
- Why subscribe?
- About Packt
- Python Machine Learning By Example Second Edition
- Copyright and Credits
- Title Page
- coverpage
- coverpage
- Title Page
- Copyright and Credits
- Python Machine Learning By Example Second Edition
- About Packt
- Why subscribe?
- Packt.com
- Dedication
- Foreword
- Contributors
- About the author
- About the reviewer
- Packt is searching for authors like you
- Preface
- Who this book is for
- What this book covers
- To get the most out of this book
- Download the example code files
- Download the color images
- Conventions used
- Get in touch
- Reviews
- Section 1: Fundamentals of Machine Learning
- Getting Started with Machine Learning and Python
- Defining machine learning and why we need it
- A very high-level overview of machine learning technology
- Types of machine learning tasks
- A brief history of the development of machine learning algorithms
- Core of machine learning – generalizing with data
- Overfitting underfitting and the bias-variance trade-off
- Avoiding overfitting with cross-validation
- Avoiding overfitting with regularization
- Avoiding overfitting with feature selection and dimensionality reduction
- Preprocessing exploration and feature engineering
- Missing values
- Label encoding
- One hot encoding
- Scaling
- Polynomial features
- Power transform
- Binning
- Combining models
- Voting and averaging
- Bagging
- Boosting
- Stacking
- Installing software and setting up
- Setting up Python and environments
- Installing the various packages
- NumPy
- SciPy
- Pandas
- Scikit-learn
- TensorFlow
- Summary
- Exercises
- Section 2: Practical Python Machine Learning By Example
- Exploring the 20 Newsgroups Dataset with Text Analysis Techniques
- How computers understand language - NLP
- Picking up NLP basics while touring popular NLP libraries
- Corpus
- Tokenization
- PoS tagging
- Named-entity recognition
- Stemming and lemmatization
- Semantics and topic modeling
- Getting the newsgroups data
- Exploring the newsgroups data
- Thinking about features for text data
- Counting the occurrence of each word token
- Text preprocessing
- Dropping stop words
- Stemming and lemmatizing words
- Visualizing the newsgroups data with t-SNE
- What is dimensionality reduction?
- t-SNE for dimensionality reduction
- Summary
- Exercises
- Mining the 20 Newsgroups Dataset with Clustering and Topic Modeling Algorithms
- Learning without guidance – unsupervised learning
- Clustering newsgroups data using k-means
- How does k-means clustering work?
- Implementing k-means from scratch
- Implementing k-means with scikit-learn
- Choosing the value of k
- Clustering newsgroups data using k-means
- Discovering underlying topics in newsgroups
- Topic modeling using NMF
- Topic modeling using LDA
- Summary
- Exercises
- Detecting Spam Email with Naive Bayes
- Getting started with classification
- Types of classification
- Applications of text classification
- Exploring Na?ve Bayes
- Learning Bayes' theorem by examples
- The mechanics of Na?ve Bayes
- Implementing Na?ve Bayes from scratch
- Implementing Na?ve Bayes with scikit-learn
- Classification performance evaluation
- Model tuning and cross-validation
- Summary
- Exercise
- Classifying Newsgroup Topics with Support Vector Machines
- Finding separating boundary with support vector machines
- Understanding how SVM works through different use cases
- Case 1 – identifying a separating hyperplane
- Case 2 – determining the optimal hyperplane
- Case 3 – handling outliers
- Implementing SVM
- Case 4 – dealing with more than two classes
- The kernels of SVM
- Case 5 – solving linearly non-separable problems
- Choosing between linear and RBF kernels
- Classifying newsgroup topics with SVMs
- More example – fetal state classification on cardiotocography
- A further example – breast cancer classification using SVM with TensorFlow
- Summary
- Exercise
- Predicting Online Ad Click-Through with Tree-Based Algorithms
- Brief overview of advertising click-through prediction
- Getting started with two types of data – numerical and categorical
- Exploring decision tree from root to leaves
- Constructing a decision tree
- The metrics for measuring a split
- Implementing a decision tree from scratch
- Predicting ad click-through with decision tree
- Ensembling decision trees – random forest
- Implementing random forest using TensorFlow
- Summary
- Exercise
- Predicting Online Ad Click-Through with Logistic Regression
- Converting categorical features to numerical – one-hot encoding and ordinal encoding
- Classifying data with logistic regression
- Getting started with the logistic function
- Jumping from the logistic function to logistic regression
- Training a logistic regression model
- Training a logistic regression model using gradient descent
- Predicting ad click-through with logistic regression using gradient descent
- Training a logistic regression model using stochastic gradient descent
- Training a logistic regression model with regularization
- Training on large datasets with online learning
- Handling multiclass classification
- Implementing logistic regression using TensorFlow
- Feature selection using random forest
- Summary
- Exercises
- Scaling Up Prediction to Terabyte Click Logs
- Learning the essentials of Apache Spark
- Breaking down Spark
- Installing Spark
- Launching and deploying Spark programs
- Programming in PySpark
- Learning on massive click logs with Spark
- Loading click logs
- Splitting and caching the data
- One-hot encoding categorical features
- Training and testing a logistic regression model
- Feature engineering on categorical variables with Spark
- Hashing categorical features
- Combining multiple variables – feature interaction
- Summary
- Exercises
- Stock Price Prediction with Regression Algorithms
- Brief overview of the stock market and stock prices
- What is regression?
- Mining stock price data
- Getting started with feature engineering
- Acquiring data and generating features
- Estimating with linear regression
- How does linear regression work?
- Implementing linear regression
- Estimating with decision tree regression
- Transitioning from classification trees to regression trees
- Implementing decision tree regression
- Implementing regression forest
- Estimating with support vector regression
- Implementing SVR
- Estimating with neural networks
- Demystifying neural networks
- Implementing neural networks
- Evaluating regression performance
- Predicting stock price with four regression algorithms
- Summary
- Exercise
- Section 3: Python Machine Learning Best Practices
- Machine Learning Best Practices
- Machine learning solution workflow
- Best practices in the data preparation stage
- Best practice 1 – completely understanding the project goal
- Best practice 2 – collecting all fields that are relevant
- Best practice 3 – maintaining the consistency of field values
- Best practice 4 – dealing with missing data
- Best practice 5 – storing large-scale data
- Best practices in the training sets generation stage
- Best practice 6 – identifying categorical features with numerical values
- Best practice 7 – deciding on whether or not to encode categorical features
- Best practice 8 – deciding on whether or not to select features and if so how to do so
- Best practice 9 – deciding on whether or not to reduce dimensionality and if so how to do so
- Best practice 10 – deciding on whether or not to rescale features
- Best practice 11 – performing feature engineering with domain expertise
- Best practice 12 – performing feature engineering without domain expertise
- Best practice 13 – documenting how each feature is generated
- Best practice 14 – extracting features from text data
- Best practices in the model training evaluation and selection stage
- Best practice 15 – choosing the right algorithm(s) to start with
- Na?ve Bayes
- Logistic regression
- SVM
- Random forest (or decision tree)
- Neural networks
- Best practice 16 – reducing overfitting
- Best practice 17 – diagnosing overfitting and underfitting
- Best practice 18 – modeling on large-scale datasets
- Best practices in the deployment and monitoring stage
- Best practice 19 – saving loading and reusing models
- Best practice 20 – monitoring model performance
- Best practice 21 – updating models regularly
- Summary
- Exercises
- Other Books You May Enjoy
- Leave a review - let other readers know what you think 更新時間:2021-07-02 12:42:13