舉報(bào)
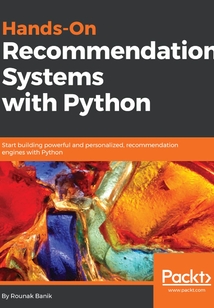
會(huì)員
Hands-On Recommendation Systems with Python
Recommendationsystemsareattheheartofalmosteveryinternetbusinesstoday;fromFacebooktoNet?ixtoAmazon.Providinggoodrecommendations,whetherit'sfriends,movies,orgroceries,goesalongwayindefininguserexperienceandenticingyourcustomerstouseyourplatform.Thisbookshowsyouhowtodojustthat.YouwilllearnaboutthedifferentkindsofrecommendersusedintheindustryandseehowtobuildthemfromscratchusingPython.Noneedtowadethroughtonsofmachinelearningtheory—you'llgetstartedwithbuildingandlearningaboutrecommendersasquicklyaspossible..Inthisbook,youwillbuildanIMDBTop250clone,acontent-basedenginethatworksonmoviemetadata.You'llusecollaborativefilterstomakeuseofcustomerbehaviordata,andaHybridRecommenderthatincorporatescontentbasedandcollaborativefilteringtechniquesWiththisbook,allyouneedtogetstartedwithbuildingrecommendationsystemsisafamiliaritywithPython,andbythetimeyou'refnished,youwillhaveagreatgraspofhowrecommendersworkandbeinastrongpositiontoapplythetechniquesthatyouwilllearntoyourownproblemdomains.
目錄(132章)
倒序
- 封面
- Title Page
- Copyright and Credits
- Hands-On Recommendation Systems with Python
- Dedication
- Packt Upsell
- Why subscribe?
- PacktPub.com
- Contributors
- About the author
- About the reviewer
- Image credits
- Packt is searching for authors like you
- Preface
- Who this book is for
- What this book covers
- To get the most out of this book
- Download the example code files
- Download the color images
- Code in action
- Conventions used
- Get in touch
- Reviews
- Getting Started with Recommender Systems
- Technical requirements
- What is a recommender system?
- The prediction problem
- The ranking problem
- Types of recommender systems
- Collaborative filtering
- User-based filtering
- Item-based filtering
- Shortcomings
- Content-based systems
- Knowledge-based recommenders
- Hybrid recommenders
- Summary
- Manipulating Data with the Pandas Library
- Technical requirements
- Setting up the environment
- The Pandas library
- The Pandas DataFrame
- The Pandas Series
- Summary
- Building an IMDB Top 250 Clone with Pandas
- Technical requirements
- The simple recommender
- The metric
- The prerequisties
- Calculating the score
- Sorting and output
- The knowledge-based recommender
- Genres
- The build_chart function
- Summary
- Building Content-Based Recommenders
- Technical requirements
- Exporting the clean DataFrame
- Document vectors
- CountVectorizer
- TF-IDFVectorizer
- The cosine similarity score
- Plot description-based recommender
- Preparing the data
- Creating the TF-IDF matrix
- Computing the cosine similarity score
- Building the recommender function
- Metadata-based recommender
- Preparing the data
- The keywords and credits datasets
- Wrangling keywords cast and crew
- Creating the metadata soup
- Generating the recommendations
- Suggestions for improvements
- Summary
- Getting Started with Data Mining Techniques
- Problem statement
- Similarity measures
- Euclidean distance
- Pearson correlation
- Cosine similarity
- Clustering
- k-means clustering
- Choosing k
- Other clustering algorithms
- Dimensionality reduction
- Principal component analysis
- Other dimensionality reduction techniques
- Linear-discriminant analysis
- Singular value decomposition
- Supervised learning
- k-nearest neighbors
- Classification
- Regression
- Support vector machines
- Decision trees
- Ensembling
- Bagging and random forests
- Boosting
- Evaluation metrics
- Accuracy
- Root mean square error
- Binary classification metrics
- Precision
- Recall
- F1 score
- Summary
- Building Collaborative Filters
- Technical requirements
- The framework
- The MovieLens dataset
- Downloading the dataset
- Exploring the data
- Training and test data
- Evaluation
- User-based collaborative filtering
- Mean
- Weighted mean
- User demographics
- Item-based collaborative filtering
- Model-based approaches
- Clustering
- Supervised learning and dimensionality reduction
- Singular-value decomposition
- Summary
- Hybrid Recommenders
- Technical requirements
- Introduction
- Case study – Building a hybrid model
- Summary
- Other Books You May Enjoy
- Leave a review - let other readers know what you think 更新時(shí)間:2021-07-16 18:19:27
推薦閱讀
- 計(jì)算機(jī)應(yīng)用
- 網(wǎng)上沖浪
- 程序設(shè)計(jì)缺陷分析與實(shí)踐
- ServiceNow Cookbook
- JMAG電機(jī)電磁仿真分析與實(shí)例解析
- 計(jì)算機(jī)網(wǎng)絡(luò)技術(shù)基礎(chǔ)
- 可編程序控制器應(yīng)用實(shí)訓(xùn)(三菱機(jī)型)
- Dreamweaver CS6精彩網(wǎng)頁(yè)制作與網(wǎng)站建設(shè)
- Windows安全指南
- 基于Proteus的單片機(jī)應(yīng)用技術(shù)
- 與人共融機(jī)器人的關(guān)節(jié)力矩測(cè)量技術(shù)
- 計(jì)算機(jī)組裝與維修實(shí)訓(xùn)
- 常用傳感器技術(shù)及應(yīng)用(第2版)
- Effective Business Intelligence with QuickSight
- Windows 7來(lái)了
- 工廠電氣控制設(shè)備
- 巧學(xué)活用Photoshop
- 數(shù)據(jù)結(jié)構(gòu)與算法(C++語(yǔ)言版)
- R:Predictive Analysis
- 系統(tǒng)與服務(wù)監(jiān)控技術(shù)實(shí)踐
- Machine Learning with R Quick Start Guide
- 智能機(jī)器人制作完全手冊(cè)(第2版)
- 微機(jī)原理與接口技術(shù)(基于32位機(jī))
- Prezi Essentials
- 深度學(xué)習(xí):語(yǔ)音識(shí)別技術(shù)實(shí)踐
- Fedora 31 Essentials
- 計(jì)算機(jī)導(dǎo)論與C語(yǔ)言
- R Web Scraping Quick Start Guide
- ARM嵌入式系統(tǒng)技術(shù)開(kāi)發(fā)與應(yīng)用實(shí)踐
- Mastering PostgreSQL 12