舉報
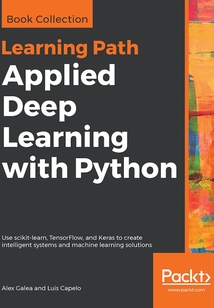
會員
Applied Deep Learning with Python
TakinganapproachthatusesthelatestdevelopmentsinthePythonecosystem,you’llfirstbeguidedthroughtheJupyterecosystem,keyvisualizationlibrariesandpowerfuldatasanitizationtechniquesbeforewetrainourfirstpredictivemodel.We’llexploreavarietyofapproachestoclassificationlikesupportvectornetworks,randomdecisionforestsandk-nearestneighbourstobuildoutyourunderstandingbeforewemoveintomorecomplexterritory.It’sokayifthesetermsseemoverwhelming;we’llshowyouhowtoputthemtowork.We’llbuilduponourclassificationcoveragebytakingaquicklookatethicalwebscrapingandinteractivevisualizationstohelpyouprofessionallygatherandpresentyouranalysis.It’safterthisthatwestartbuildingoutourkeystonedeeplearningapplication,onethataimstopredictthefuturepriceofBitcoinbasedonhistoricalpublicdata.Byguidingyouthroughatrainedneuralnetwork,we’llexplorecommondeeplearningnetworkarchitectures(convolutional,recurrent,generativeadversarial)andbranchoutintodeepreinforcementlearningbeforewediveintomodeloptimizationandevaluation.We’lldoallofthiswhilstworkingonaproduction-readywebapplicationthatcombinesTensorflowandKerastoproduceameaningfuluser-friendlyresult,leavingyouwithalltheskillsyouneedtotackleanddevelopyourownreal-worlddeeplearningprojectsconfidentlyandeffectively.
目錄(166章)
倒序
- 封面
- Title Page
- Copyright and Credits
- Applied Deep Learning with Python
- Packt Upsell
- Why subscribe?
- Packt.com
- Contributors
- About the authors
- About the reviewers
- Packt is searching for authors like you
- Preface
- Who this book is for
- What this book covers
- To get the most out of this book
- Download the example code files
- Conventions used
- Get in touch
- Reviews
- Jupyter Fundamentals
- Basic Functionality and Features
- What is a Jupyter Notebook and Why is it Useful?
- Navigating the Platform
- Introducing Jupyter Notebooks
- Jupyter Features
- Exploring some of Jupyter's most useful features
- Converting a Jupyter Notebook to a Python Script
- Python Libraries
- Import the external libraries and set up the plotting environment
- Our First Analysis - The Boston Housing Dataset
- Loading the Data into Jupyter Using a Pandas DataFrame
- Load the Boston housing dataset
- Data Exploration
- Explore the Boston housing dataset
- Introduction to Predictive Analytics with Jupyter Notebooks
- Linear models with Seaborn and scikit-learn
- Activity: Building a Third-Order Polynomial Model
- Linear models with Seaborn and scikit-learn
- Using Categorical Features for Segmentation Analysis
- Create categorical fields from continuous variables and make segmented visualizations
- Summary
- Data Cleaning and Advanced Machine Learning
- Preparing to Train a Predictive Model
- Determining a Plan for Predictive Analytics
- Preprocessing Data for Machine Learning
- Exploring data preprocessing tools and methods
- Activity: Preparing to Train a Predictive Model for the Employee-Retention Problem
- Training Classification Models
- Introduction to Classification Algorithms
- Training two-feature classification models with scikit-learn
- The plot_decision_regions Function
- Training k-nearest neighbors for our model
- Training a Random Forest
- Assessing Models with k-Fold Cross-Validation and Validation Curves
- Using k-fold cross-validation and validation curves in Python with scikit-learn
- Dimensionality Reduction Techniques
- Training a predictive model for the employee retention problem
- Summary
- Web Scraping and Interactive Visualizations
- Scraping Web Page Data
- Introduction to HTTP Requests
- Making HTTP Requests in the Jupyter Notebook
- Handling HTTP requests with Python in a Jupyter Notebook
- Parsing HTML in the Jupyter Notebook
- Parsing HTML with Python in a Jupyter Notebook
- Activity: Web Scraping with Jupyter Notebooks
- Interactive Visualizations
- Building a DataFrame to Store and Organize Data
- Building and merging Pandas DataFrames
- Introduction to Bokeh
- Introduction to interactive visualizations with Bokeh
- Activity: Exploring Data with Interactive Visualizations
- Summary
- Introduction to Neural Networks and Deep Learning
- What are Neural Networks?
- Successful Applications
- Why Do Neural Networks Work So Well?
- Representation Learning
- Function Approximation
- Limitations of Deep Learning
- Inherent Bias and Ethical Considerations
- Common Components and Operations of Neural Networks
- Configuring a Deep Learning Environment
- Software Components for Deep Learning
- Python 3
- TensorFlow
- Keras
- TensorBoard
- Jupyter Notebooks Pandas and NumPy
- Activity: Verifying Software Components
- Exploring a Trained Neural Network
- MNIST Dataset
- Training a Neural Network with TensorFlow
- Training a Neural Network
- Testing Network Performance with Unseen Data
- Activity: Exploring a Trained Neural Network
- Summary
- Model Architecture
- Choosing the Right Model Architecture
- Common Architectures
- Convolutional Neural Networks
- Recurrent Neural Networks
- Generative Adversarial Networks
- Deep Reinforcement Learning
- Data Normalization
- Z-score
- Point-Relative Normalization
- Maximum and Minimum Normalization
- Structuring Your Problem
- Activity: Exploring the Bitcoin Dataset and Preparing Data for Model
- Using Keras as a TensorFlow Interface
- Model Components
- Activity: Creating a TensorFlow Model Using Keras
- From Data Preparation to Modeling
- Training a Neural Network
- Reshaping Time-Series Data
- Making Predictions
- Overfitting
- Activity: Assembling a Deep Learning System
- Summary
- Model Evaluation and Optimization
- Model Evaluation
- Problem Categories
- Loss Functions Accuracy and Error Rates
- Different Loss Functions Same Architecture
- Using TensorBoard
- Implementing Model Evaluation Metrics
- Evaluating the Bitcoin Model
- Overfitting
- Model Predictions
- Interpreting Predictions
- Activity:Creating an Active Training Environment
- Hyperparameter Optimization
- Layers and Nodes - Adding More Layers
- Adding More Nodes
- Layers and Nodes - Implementation
- Epochs
- Epochs - Implementation
- Activation Functions
- Linear (Identity)
- Hyperbolic Tangent (Tanh)
- Rectifid Linear Unit
- Activation Functions - Implementation
- Regularization Strategies
- L2 Regularization
- Dropout
- Regularization Strategies – Implementation
- Optimization Results
- Activity:Optimizing a Deep Learning Model
- Summary
- Productization
- Handling New Data
- Separating Data and Model
- Data Component
- Model Component
- Dealing with New Data
- Re-Training an Old Model
- Training a New Model
- Activity: Dealing with New Data
- Deploying a Model as a Web Application
- Application Architecture and Technologies
- Deploying and Using Cryptonic
- Activity: Deploying a Deep Learning Application
- Summary
- Other Books You May Enjoy
- Leave a review - let other readers know what you think 更新時間:2021-08-13 15:53:42
推薦閱讀
- LabVIEW 2018 虛擬儀器程序設計
- Power Up Your PowToon Studio Project
- PHP 從入門到項目實踐(超值版)
- C#程序設計基礎:教程、實驗、習題
- Mastering Linux Network Administration
- Hands-On Natural Language Processing with Python
- Instant Ext.NET Application Development
- Mastering Unity 2D Game Development(Second Edition)
- 智能手機APP UI設計與應用任務教程
- Visual Studio 2015高級編程(第6版)
- Kivy Cookbook
- 響應式Web設計:HTML5和CSS3實戰(第2版)
- Instant jQuery Boilerplate for Plugins
- Android Development Tools for Eclipse
- C++程序設計教程
- Photoshop智能手機APP界面設計
- 軟硬件綜合系統軟件需求建模及可靠性綜合試驗、分析、評價技術
- Developer,Advocate!
- GO語言編程從入門到實踐
- Kudu:構建高性能實時數據分析存儲系統
- Learn Spring for Android Application Development
- C語言開發入門教程
- 零基礎學C++
- Android for the BeagleBone Black
- 深入理解Go語言
- Java EE企業級應用開發技術研究
- OpenCV計算機視覺編程攻略(第3版)
- Unity 3D特效設計必修課
- Node.js權威指南
- C#編程寶典(十年典藏版)